The rise of artificial intelligence: benefits and risks for financial stability
Published as part of the Financial Stability Review, May 2024.
The emergence of generative artificial intelligence (AI) tools represents a significant technological leap forward, with the potential to have a substantial impact on the financial system. Conceptually, AI brings both benefits and risks to the financial system. Practically, the overall impact will depend on how the challenges related to data, model development and deployment are addressed – both at the level of financial institutions and for the financial system as a whole. If new AI tools are used widely in the financial system and AI suppliers are concentrated, operational risk (including cyber risk), market concentration and too-big-to-fail externalities may increase. Furthermore, widespread AI adoption may harbour the potential for increased herding behaviour and market correlation. Should concerns arise that cannot be tackled by the current regulatory framework, targeted initiatives may need to be considered.
Introduction
Since late 2022 public interest in AI has increased sharply, and the volume of AI-related jobs, innovations and patents is growing constantly. Google searches for AI-related terms have surged since the launch of ChatGPT. At the same time, the number of AI-related jobs, AI models and patents connected to AI is growing constantly. Most of the recently launched models are language or multimodal models, and in recent years Europe has had more people working in AI-related roles than the United States (Chart B.1). According to a recent study, 64% of businesses believe that AI will increase their productivity, while 40% of business owners are concerned about technology dependence.[2] Other estimates show that among industries globally, generative AI could add the equivalent of between USD 2.6 trillion and USD 4.4 trillion of economic value annually. Banking is expected to be a large beneficiary.[3],[4]
Chart B.1
Attention on AI has spiked and AI-related jobs, innovations and patents are increasing
a) Professionals employed in AI roles, by region, and AI-related Google search trends | b) Number of notable AI systems and sum of AI and machine learning patents of the largest patent owners |
---|---|
(Jan. 2014-Dec. 2023; thousands, index) | (2014-23; AI systems in absolute numbers and patents in thousands) |
![]() | ![]() |
Sources: Google Trends, State of European Tech*, Epoch**, Statista*** and ECB calculations.
Notes: Panel a: Google search trends represent monthly global search interest relative to the highest point, indicated by the value 100 and for each search term. Figures on AI jobs comprise all companies, non-tech included, based on an analysis of the job titles of 216 million professionals. The universe of professionals considered to be actively employed in AI/ML roles is based on a search utilising both common job titles in the field (e.g. AI researcher, ML engineer) and key phrases used in job titles (e.g. deep learning). Panel b: patents are cumulative over the ten largest patent owners (one South Korean, two US and seven Chinese companies and institutions) in machine learning and AI worldwide from 2013 to 2022 and are lagged by one year in the plot “Number of notable AI systems”. The authors of the Epoch dataset have established a set of criteria used to identify key AI systems which they refer to as “notable”. Such systems must demonstrate the ability to learn, show tangible experimental results and contribute advancements that push the boundaries of existing AI technology. In terms of notability, the AI must have garnered extensive academic attention, evidenced by a high citation count, hold historical significance in the field, mark a substantial advancement in technology or be implemented in a significant real-world context. The authors recognise the difficulty of evaluating the impact of newer AI systems since 2020 given the fact that less data are available for the period. They therefore also employ subjective judgement when selecting recent developments.
*) “Embrace risk, shape the future”, State of European Tech, 2023.
**) Epoch (2024) – with minor processing by Our World in Data. “Annual number of AI systems by domain” [dataset]. Epoch, “Parameter, Compute and Data Trends in Machine Learning” [original data].
***) Wunsch, N.-G., “Companies with the most machine learning & AI patents worldwide 2013-2022”, Statista, April 2023.
The pace and scale of AI, like any sweeping innovation, is likely to bring benefits but could also pose risks for financial stability. International standard-setters and regulatory authorities have intensified their efforts regarding the consequences of AI for the financial system.[5] There is a broad consensus that the use of AI is associated with possible benefits for numerous sectors, including the financial sector. It is therefore no surprise that euro area banks are exploring and using innovative technologies such as AI to support their digital transformation (Chart B.2, panel b). At the same time, there could also be AI risks for financial institutions and, potentially, the wider financial system.
This special feature provides a conceptual framework for assessing the systemic implications of AI for the financial system. To this end, the feature first investigates how the benefits and risks for individual financial institutions using AI are related to the technological aspects of AI. Next, it assesses how these benefits and risks at the firm level could unfold at the macro level, potentially leading to implications for financial stability.
What is AI?
AI is a broad term including various sub-fields and technological concepts. AI comprises two broad strands (Figure B.1)[6]: (1) data-driven machine learning systems; and (2) rule-based approaches such as deterministic chatbots, built on if/else instructions. Machine learning contains traditional statistical models and artificial neural networks. A hallmark of such networks is that they aim to replicate the learning process of the human brain. These models can capture non-linear properties of data and apply previously gained knowledge to new problems. Recently, the capabilities of artificial neural networks have been significantly boosted by increasing their complexity and training them on a vast amount of data. The rise of this new class of models, generally called foundation models,[7] was mainly enabled by the decreasing cost and increasing efficiency of computational power.[8]
Foundation models form a knowledge base for generative AI. These models are “trained” in a self-supervised[9] manner on a vast amount of both structured (e.g. tables) and unstructured (images, sound, text) raw data with only minimal human intervention. In the pre-training phase, the model learns the fundamental structure (“ground truth”) of the data in a generic way, covering aspects like use of human language, recognition of objects and images, and numerical input. Generative AI models can make use of the generic knowledge of foundation models.[10] A key feature of generative AI is its ability to produce unique output in the form of text, images and audio which share some properties of the input data but differ in others (generative capabilities). Most current generative AI models are based on text (large language models, or LLMs), thus eliminating the need for proficient coding skills to modify or use them. The performance of foundation models can be enhanced by providing additional training on task-related data (fine-tuning) or by embedding additional tools like search engines.
Figure B.1
Systematic overview of AI and sub-fields
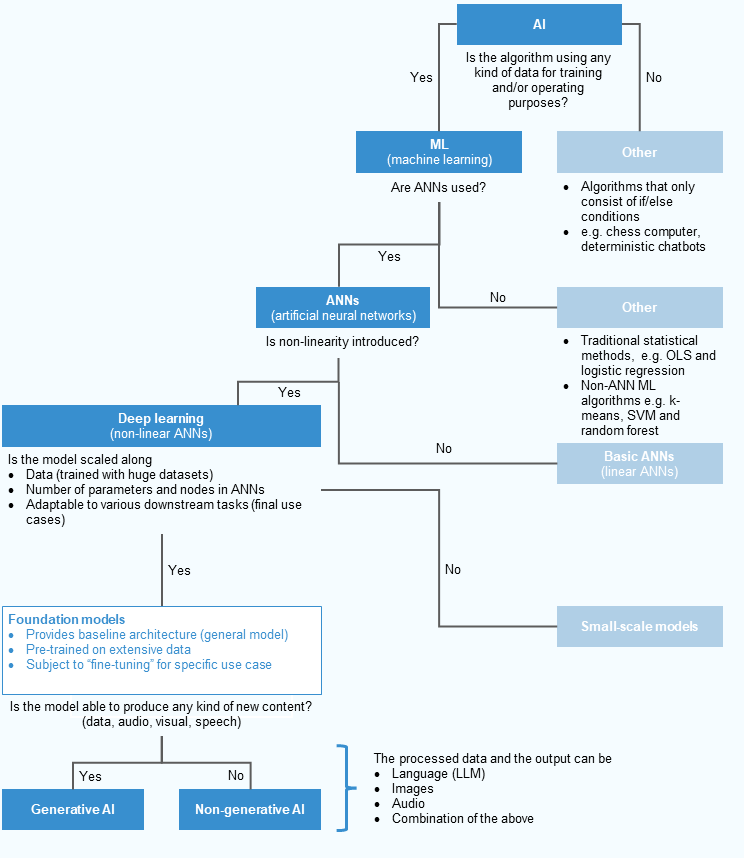
Source: ECB analysis.
Notes: This chart shows a possible systematic overview of AI and its sub-fields to facilitate understanding and distinguish different sub-fields of AI. Although its purpose is to facilitate an understanding and an evaluation of the impact of AI on financial stability, the overview could be extended to a more granular level for other use cases (e.g. distinguishing AI in machine learning, robotics, vision etc.) ).There is no clear scientific taxonomy of AI and its sub-fields at this stage, but this chart represents a possible classification, which is in line with scientific discussions and approaches. For the distinction between AI and machine learning, see Das, S. et al. “Applications of Artificial Intelligence in Machine Learning: Review and Prospect”, International Journal of Computer Applications, Vol. 115, No 9, 2015. The paths from machine learning, artificial neural networks (ANNs) and deep learning mostly follow the definition found in Montesinos, L. et al., “Fundamentals of Artificial Neural Networks and Deep Learning”, Multivariate Statistical Machine Learning Methods for Genomic Prediction, Springer, Cham, 2022. For the definition of foundation models and their connection to generative AI, see, for example, Bommasani, R. et al., “On the Opportunities and Risks of Foundation Models”, ArXiv, 2021.
Henceforth, when discussing AI we will generally refer to foundation models and generative AI. Foundation models and the generative AI models based on such models add new aspects to consider when assessing implications for the financial system. Therefore, this discussion focuses explicitly on these models.
Although AI has made significant progress, its cognitive limits should be acknowledged. Generative AI models have been referred to as “stochastic parrots”.[11] The language they generate is often hard to distinguish from human interaction, yet in essence it is the outcome of a stochastic process that combines text based on probabilistic information. The term artificial intelligence may thus be a misnomer as it suggests “intelligence”, whereas in fact the model does not fundamentally understand the underlying logic of the text.[12]
Benefits and risks of AI for financial institutions
It is challenging to establish a comprehensive assessment of the implications of AI for the financial system as the technology is still evolving. Accordingly, any discussions of the benefits, risks and systemic consequences of AI are largely based on conjecture. That said, a preliminary view can be drawn from the latest trends, concepts and debates in publications, industry reports and ECB market intelligence reports.[13]
The benefits and risks of AI depend on the use case. The development and deployment cycle (Figure B.2) establishes a conceptual framework for a structured assessment of the benefits and risks stemming from AI at the level of individual financial firm. Three main building blocks are required to apply AI to a specific use case: training data, the model itself and the deployment or implementation of the tool.
Although AI greatly enhances the processing and generation of data, it may be prone to significant data quality issues. AI systems based on foundation models can process and analyse unstructured data beyond numerical input. These data include text, computer code, voice and images. AI can also be used to manage and create data.[14] However, the way foundation models are trained means that they may be more likely to “learn” and sustain biases or errors inherent in the data they have been trained on. Hence, foundation models may be prone to data quality issues.[15] One additional challenge concerns data privacy, notably whether publicly available systems respect user input data privacy (which could, for instance, also be confidential firm-specific information) and whether there is a risk of data leakage.
Figure B.2
The AI development and deployment cycle – a conceptual framework for a structured assessment of the benefits and risks to financial system
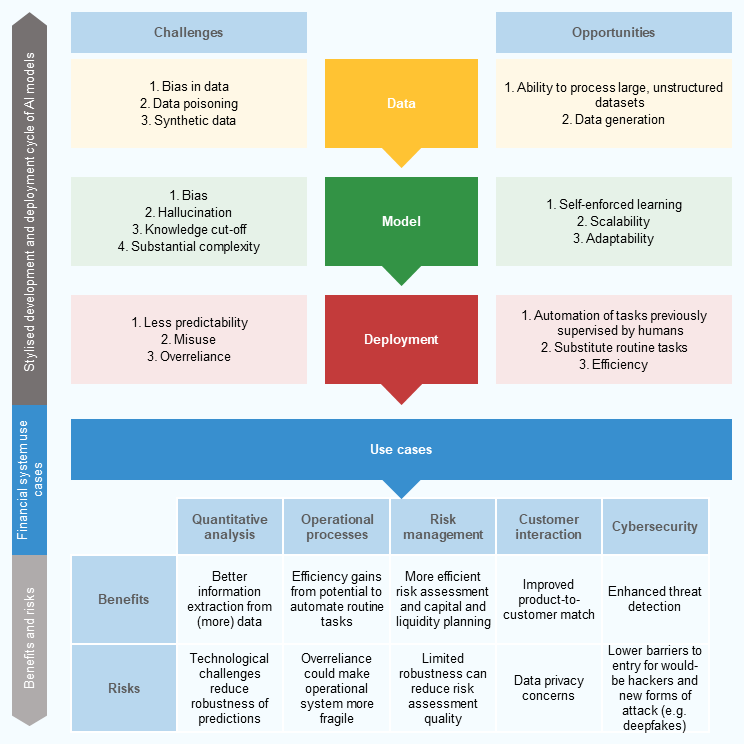
Source: ECB analysis.
Notes: The first part of Figure B.2 describes the different phases in the development and deployment of an AI system, mentioning possible opportunities and challenges. opportunities and challenges are inherited throughout the phases and only take specific form in terms of benefits and risks, depending on the final use case. The table showing use-case-specific benefits and risks could change in the future, depending on technological developments and how institutions use the technology.
AI models are adaptable, flexible and scalable, but prone to bias, hallucination and greater complexity, which makes them less robust. The general-purpose base architecture of AI can be fine-tuned to perform more specialised tasks. This can be achieved by training the model on specific data, for instance. This feature significantly enhances a model’s capabilities in a targeted area while retaining its overall generative capabilities. AI is thus adaptable and scalable for different use cases.[16] That said, AI is prone to algorithmic bias, whereby the model systematically favours certain outcomes which have inequitable results. It may also present false or misleading information as facts – known as “hallucinations”.[17] As recent AI models are much more complicated than traditional models, it is very difficult for humans to comprehend and reconstruct the predictions made.[18] Furthermore, as AI may not be trained on the most recent information available, its capabilities may be limited by a technological knowledge cut-off. Together, these challenges strongly limit the robustness of AI predictions.
When deployed, AI can increase efficiency, but its performance is difficult to predict and subject to possible misuse or overreliance. Thanks to AI’s inherent flexibility, it is expected that financial institutions will be able to deploy AI tools in a large variety of use cases, including tasks that have so far been performed by human labour. This is likely to result in greater efficiency and significant cost savings. At the same time, such deployment in new tasks and processes presents a risk, as it is difficult to predict and control ex ante how AI will perform in practice. AI systems can develop unexpected, potentially harmful capabilities when applied to new use cases.[19] Furthermore, it is not inconceivable that AI could be misused in a harmful manner. For example, criminals could fine-tune and spoil otherwise harmless AI for specific operations (e.g. cyberattacks, misinformation, market manipulation, use of deep fakes to undermine confidence in a financial institution, etc.), increasing their threat potential.
Financial institutions can be expected to deploy AI in several ways. In view of the enhanced capabilities of AI and the wealth of data available for financial institutions from which predictions can be made or new information generated, AI models could be usefully deployed in quantitative analysis, operational processes, risk management, client interaction and cybersecurity, among other areas. Given the rapid developments in these areas, the suggested conceptual framework does not exclude possible further use cases or alternative classifications.
AI may improve the processing of information and the accuracy of quantitative predictions, but the robustness of its predictions remains a challenge. AI’s flexibility in analysing various forms of input data, together with its generative and predictive capabilities, will allow financial institutions to use it for data management, data creation and assessment functions. As such, AI could be used to systematically extract and prepare information in real time from various sources simultaneously (media, industry reports, conversations, market data, etc.) that can be used to form predictions. This could significantly improve the available information, leading to more precise decision-making and hence better outcomes (e.g. in trading, asset allocation, etc.). However, hallucination, algorithmic bias and vulnerability to data quality issues present risks to the accuracy of AI predictions. If financial entities base their decisions on faulty AI predictions which are not checked, this could lead to outcomes that may result in economic losses or even disorderly market moves. Furthermore, the complexity of AI could make it difficult to identify the root cause of errors or explain and justify any decision based on AI.[20]
AI may improve the efficiency of financial institutions’ operational processes, but operational risk and third-party dependence may increase. AI could be applied in various internal operational processes. These could range from co-piloting functions that automatically proofread or complete drafting text or coding, to more sophisticated algorithms (e.g. chatbots or digital assistants) that can automate routine tasks or entire workstreams.[21] These applications would free up human resources, improve cost structures and potentially reduce human-induced error. On the other hand, data-, model- and deployment-related challenges may undermine AI’s robustness and, if AI is used to back up critical operational processes, this could significantly increase operational risk. Furthermore, depending on whether financial institutions have the in-house capacity to develop foundation models, the base architecture may need to be acquired from external companies. This will increase third-party reliance and could also raise data privacy concerns if the models provided by third parties are fine-tuned using confidential internal data (e.g. internal records, financial statements, etc.).
AI could enhance the risk management functions of financial firms, but could also weaken them if its predictions prove unreliable. Risk management functions could be seen as sub-groups of the areas of quantitative analysis and operational processes. AI in this domain could be used for fraud detection and monitoring (e.g. for anti-money-laundering purposes), for capital and liquidity risk monitoring and planning, and for regulatory compliance.[22] The considerations that apply to any AI-based quantitative analysis in terms of expected benefits and risk similarly apply to its deployment in risk functions. AI could enhance risk management capabilities, leading to more accurate risk assessment and predictions and more efficient capital and liquidity planning. At the same time, algorithmic bias, hallucination and other challenges could make institutions’ risk assessments that rely on AI less reliable and robust. Any benefits or risks that can be implied from AI use in risk management will have direct implications for the resilience of the financial sector from a prudential point of view, necessitating close monitoring by all stakeholders, including financial institutions’ management bodies and supervisory authorities.
AI in customer-facing operations may improve the product-to-customer match, but its use could also lead to customer discrimination if it goes unchecked. It is expected that AI will unlock multiple new applications in customer-facing activities. These could be in communication, onboarding and complaints management (e.g. using automated chatbots), advisory functions (e.g. using digital assistants/robo-advisors) or for customer segmentation and targeting. AI will be able to better analyse a variety of customer-related data which could lead to better tailored products and services. This could improve financial institutions’ product-to-customer match, increasing economic efficiency for both the institution and the customer.[23] However, algorithmic bias may lead to discriminatory customer treatment and be difficult to identify and monitor. Furthermore, the issue of data leakage is particularly sensitive in the case of AI trained on customer-specific data, raising consumer protection considerations, and could also expose institutions to increased reputational or legal risk.
Financial stability implications of AI
The implications of AI for individual firms can become amplified to a systemic level through technological penetration and supplier concentration. There are two systemic amplifiers through which the implications of AI for single firms could become systemic. The first amplifier is technological penetration. If AI is widely adopted across different financial entities for an increasing number of processes and applications, more areas of the financial system will be affected by the challenges and opportunities associated with AI. The second amplifier is supplier concentration.[24] If a majority of financial institutions use the same or very similar foundation models provided by a few suppliers, it is likely that decisions based on AI will suffer from similar biases and technological challenges, and reliance on system providers will increase. The interplay between these two dimensions will determine whether or not the benefits and risks stemming from use cases at individual institution level become systemic (left side of Figure B.3).
Financial stability could be at risk if supplier concentration and technological penetration are high. On the one hand, if only a few institutions use AI and there are a large number of different providers of the technology, risks may occur at the micro level, depending on the use cases of individual institutions. On the other hand, if technological penetration and supplier concentration are high, any risk from AI that is relevant at the micro level could be amplified and lead to financial stability consequences. The transition from micro to macro could be gradual, but not necessarily linear.
Figure B.3
Systemic amplifiers of AI and financial stability challenges
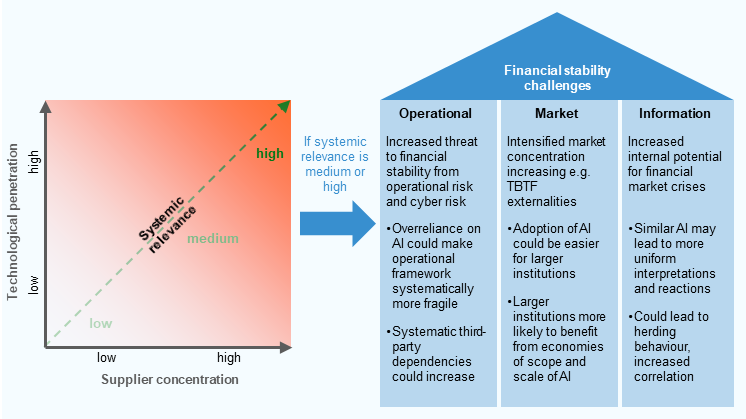
Source: ECB analysis.
Notes: The left side of the chart shows how the interplay between the widespread use of AI (technological penetration) and supplier concentration of AI models can raise institution-level benefits and risks, as described in Figure B.2, to a systemic level. Different combinations result in different levels of systemic relevance. Systemic relevance increases with technological penetration and supplier concentration, but not necessarily on a straight-line basis. For illustration purposes, it has been shown as linear here. If systemic relevance is given, then institution-specific benefits and risks can result in financial stability challenges, which can broadly be categorised into three buckets. Given the future technological development and use of AI by financial institutions, other financial stability consequences could arise through these three main channels. The risks listed above may be even larger for proprietary and non-auditable systems.
Overreliance and a limited number of AI suppliers may make the operational backbone of the financial system more fragile. To leverage potential efficiency gains, financial institutions may increasingly substitute AI resources for human resources, potentially inducing an overreliance on AI in core functions that could render the financial system more vulnerable to inherent operational flaws and failures or cyberattacks. Both would be amplified if the number of AI suppliers is limited, as this would additionally increase the financial system’s dependency on third-party providers and introduce single-point-of-failure risks. This constitutes a potential threat to financial stability from the perspective of operational risk and cyber risk (Box A).
The widespread adoption of AI may increase market concentration in the financial services industry. The integration of AI into business structures may require large initial fixed investments and entail economic risks. It may be easier for larger firms with well-established data infrastructure and third-party networks to obtain the requisite technological knowledge and levels of data availability. Accordingly, some financial institutions may miss the transition or be unable to make the necessary investments, ending up permanently behind and dropping out of the market. Like other information technology, AI may prove to be a winner-takes-all market. AI may thus contribute to a further shift in market power amid an increasingly digitalised environment, leading to a higher concentration in the financial system, among either existing players or new players (e.g. from the technology industry). Ultimately, this could result in fewer institutions remaining on the market, accelerate too-big-to-fail externalities[25] and transfer economic rents from consumers to financial institutions.
AI may distort the information processing function of markets, increasing financial markets’ endogenous crisis potential. Conceptually, AI can be thought of as a filter through which information is gathered, analysed and assessed. The interpretation of information may become more uniform if increasingly similar models with the same embedded challenges and biases are widely used to understand financial market dynamics. As a result, AI may make market participants’ conclusions systematically biased, leading to distorted asset prices, increased correlation, herding behaviour or bubbles. Should many institutions use AI for asset allocation and rely only on a few AI providers, for example, then supply and demand for financial assets may be distorted systematically, triggering costly adjustments in markets that harm their resilience. Similarly, extensive use of AI by retail investors may result in large and similar shifts in retail trading patterns, which would increase volatility in market sentiment, trading volumes and prices.
It is difficult to predict what level of technological penetration and supplier concentration AI will reach in the financial system. Just over half of the investment in AI firms was in four companies (Chart B.2, panel a), indicating a high degree of supplier concentration. Whether AI will be widely used in the financial system will depend on the expected benefits and return on investment.[26] A survey of banks supervised by the ECB indicates that the majority of banks are already using traditional AI systems (Chart B.2, panel b).[27] [28] ECB market intelligence suggests, however, that the use of generative AI is still in the early stages of deployment. Market contacts indicate that euro area financial institutions may be slower to adopt generative AI, given the range of previously discussed risks,[29] making the decision to be an early adopter or follower more complex in finance than in other sectors, also considering potential reputational risks. In addition, the technological adoption strategy implies a complex trade-off between partnering with external suppliers (including big tech firms as opposed to smaller start-ups) and establishing in-house AI expertise. The latter may become more feasible if more AI base architecture becomes available as open source. Ultimately, it is these decisions that will determine the levels of technological penetration and supplier concentration.
Chart B.2
Investments in AI start-ups are concentrated among a few companies and European banks are already relying on traditional AI
a) Share of total private equity and venture capital raised by AI start-ups | b) Adoption rates of innovative technologies by banks (excluding generative AI) |
---|---|
(Dec. 2023) | (Q3 2022, percentages) |
![]() | ![]() |
Sources: ECB and PitchBook Data, Inc.
Notes: Panel a: shares represent the total amount of private equity and venture capital raised by individual AI start-ups to December 2023. Only AI start-ups that are actively financed with private equity or venture capital, raised at least €100 million and are classified by PitchBook as working on horizontal platforms are considered. PitchBook’s definition of horizontal platforms is as follows: “Horizontal platforms empower end users to build and deploy AI&ML algorithms across a variety of use cases. Some horizontal platforms are used to improve AI&ML algorithms but do not use AI&ML themselves.” 175 start-ups are grouped together under “Other companies”. The total capital raised also includes financing before the recent innovations around generative AI, meaning that the concentration shown can be seen as a lower limit of concentration among generative AI and foundation model suppliers, as the largest and explicitly named companies work on generative AI. Panel b: Cloud comprises migration/IT optimisation and data platforms using software-as-a-service (SaaS) solutions; AI comprises chatbots, credit scoring and algorithmic trading; DLT (distributed ledger technology) comprises trade finance (smart contracts) and settlements including custody of crypto-assets and tokenisation of traditional financial instruments. The data are drawn from the ECB’s horizontal assessment of the survey on digital transformation and the use of fintech, conducted with all banks supervised by the ECB.
Conclusion
AI may bring benefits and risks at the financial institution level as well as for the entire financial system. The significant technological leap forward in the domain of AI may be a driver of economic progress that benefits consumers, businesses and the economy as a whole. AI can increase the efficiency of financial intermediation via faster and more comprehensive information processing that supports decision-making, which may strengthen the financial system and contribute to financial stability as well. At the same time, the technological challenges associated with AI limit its robustness and increase risks related to bias, hallucinations or misuse. These may distort financial market outcomes, impair the robustness of the operational framework or systematically bias information processing and institutions’ risk management or decision-making.
The systemic implications of AI will depend on the levels of technological penetration and supplier concentration, which are difficult to predict. AI technology and its usage in the financial sector is still evolving. Furthermore, additional considerations, such as the broader macroeconomic and climate-related effects of AI as well as the moral and ethical aspects of the (mis-)use of AI, need to be explored further. The latter could have an impact on public trust in financial intermediation, which is a cornerstone of financial stability. Therefore, the implementation of AI across the financial system needs to be closely monitored as the technology evolves. Additionally, regulatory initiatives may need to be considered if market failures become apparent that cannot be tackled by the current prudential framework.[30]
Box A
The implications of artificial intelligence for cyber risk: a blessing and a curse
Cyber incidents can pose systemic threats to the financial system. Systemic events can arise if many institutions are affected at the same time (e.g. when a widely used program or service provider is involved) or an incident at one entity propagates to the broader system via financial, operational or confidence channels.[31] As digitalisation progresses, potentially driven further by the rise of artificial intelligence (AI), additional layers of interdependence between financial firms, digital service providers and software vendors may emerge and may act as propagation channels for cyber incidents. As such, there has been a marked increase in the number of cyber incidents in recent years (Chart A, panel a), with the trend picking up beyond key events like the US elections in 2016 and 2020 and the Russian invasion of Ukraine in 2022, which were likely associated with increased cyberattacks. This led to sizeable losses for the global economy and triggered a debate on the insurability of large-scale attacks and on system-wide safeguards.[32]
AI tools have been met with growing public and investor interest, including in the context of cyber risk. The number of publicly available AI models has grown substantially since the launch of ChatGPT in November 2022 (Chart A, panel b). Most of these models specialise in text processing, but a growing number are also designed for audio or visual purposes. At the same time, concerns have grown that recent advances in this technology may not only yield productivity-enhancing benefits but may also be used by cyber attackers for malicious purposes, highlighting the need for enhanced cyber defences. These aspects are mirrored by both Google search trends and the stock market performance of related sectors (Chart A, panels b and c).
Chart A
The advance of AI technology has sparked public and investor interest, including on its implications for cyber risk and cybersecurity
a) Number of publicly disclosed cyberattacks over time | b) Number of open-source AI models, by type, and Google searches for “Cyber attack and AI” | c) Stock price developments for cybersecurity and AI firms vs the broader market |
---|---|---|
(2014-23, total) | (Jan. 2021-Apr. 2024; left-hand scale: thousands, right-hand scale: index) | (6 Jan. 2017-3 May 2024, indices: 6 Jan. 2017 = 100) |
![]() | ![]() | ![]() |
Sources: University of Maryland CISSM Cyber Attacks Database, Google Trends, Hugging Face, Bloomberg Finance L.P. and ECB calculations.
Notes: Panel b: the number of open-source models uploaded to the Hugging Face platform. Open-source models without information on the model type have been excluded from this chart: as at April 2024 they accounted for 49% of all models. “Multimodal” refers to models which are capable of processing information from different modalities, including audio, images, videos and text. The Google Trends shown here are measured as the 12-month moving average of an index which takes the value 100 for the point of highest search interest for the term since 2004. Panel c: “Broad market” depicts the MSCI ACWI IMI, while “Cybersecurity firms” reflects the MSCI ACWI IMI Cyber Security Index. “Magnificent 7” comprises the stocks of Amazon, Apple, Alphabet, Nvidia, Meta, Microsoft and Tesla. This is used as a proxy for AI firms, as most of them are active in the AI field, while many firms specialised only in AI are not publicly traded.
When it comes to the interplay between AI and cyber risks, AI tools will enhance the capabilities of threat actors while also benefiting cybersecurity. From a conceptual perspective, opportunities arise for cyber defence where AI can, for instance, be useful for analysing large amounts of security signals, allowing for the real-time monitoring of network activity (Figure A). Pattern recognition can spot unusual user behaviour, which helps to enhance threat detection. This could also help mitigate insider threats – risky user behaviour could be identified and sensitive information could be blocked from leaving a financial institution’s network. Ultimately, there is potential for automated responses and risk mitigation. AI-driven productivity gains for cyber defenders can also help mitigate a shortage of cybersecurity experts, generate cost savings and optimise cyber-defence strategies. Nonetheless, cyber threats could also rise as AI may enlarge the pool of potential cyber criminals as well as victims, while also improving the efficiency and effectiveness of underlying techniques. For instance, AI models could be used to research potential target systems and victims or help with coding.[33] AI could help to significantly lower the entry barrier for would-be hackers or increase the effectiveness of professional hackers by finding vulnerabilities or helping evade detection. In addition, AI tools can be used as vehicles for an attack by manipulating the output they provide. AI tools with visual or audio output can be used to create deepfakes for social engineering attacks.
Figure A
Potential implications of AI for cyber risks
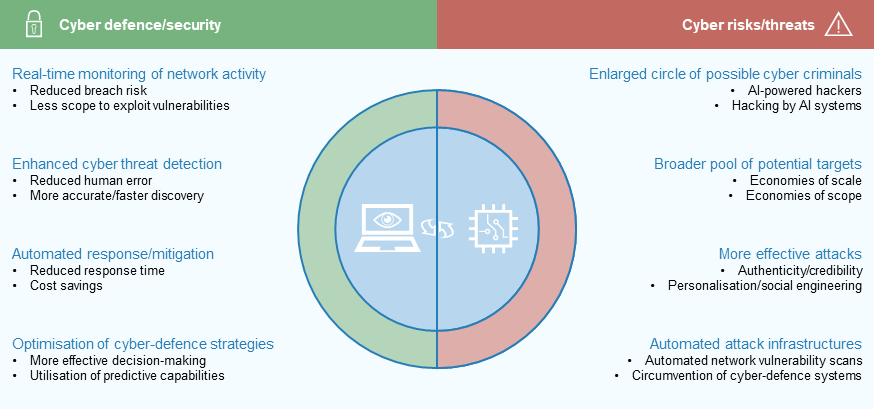
Source: ECB.
Phishing, among all types of cyberattack, seems particularly relevant for the financial industry and prone to enhancement with AI. As phishing[34] attacks rely heavily on projecting authenticity and trust, AI has a particular potential to strengthen the attacks. First, it can enhance the persuasiveness of attackers, making them sound more convincing, not just by improving written text and making it more personalised, but also by employing deepfakes for voice- or video-based communication. Second, it can automate large-scale phishing campaigns, increasing their reach and effectiveness. In fact, detected phishing activity has grown considerably in the last couple of years (Chart B, panel a), coinciding with the broader availability of AI models. These attacks target a wide range of individuals, possibly with the ultimate objective of gaining elevated or privileged level access within financial institutions’ systems (privilege escalation attack). As at year-end 2023, over a fifth of all phishing activities targeted the financial sector, making it the second most affected industry after social media (Chart B, panel a). The ensuing interlinkages are crucial not only for the financial sector but also for other industries, as information gleaned from social media profiles can often be used to gain privileged level access within an individual’s place of employment. In addition, more widespread use of social media may also help spread rumours and disinformation faster, which could raise financial stability concerns to the extent that they trigger herding behaviour.
Chart B
Phishing activity has boomed in recent years, with both financial institutions and social media increasingly targeted, highlighting the need for enhanced cyber defences
a) Total number of unique phishing websites (attacks) detected and sectoral breakdown | b) Cyber Defense Index 2022/23 |
---|---|
(left graph: 2014-23, millions; right graph: 2020, 2023, share of total number of unique phishing websites (attacks) detected) | (score from 0 (lowest) to 10 (highest)) |
![]() | ![]() |
Sources: APWG, MIT Technology Review and ECB calculations.
Notes: Panel b: “Euro area” is an unweighted average of Germany, Spain, France, Italy and the Netherlands. SaaS stands for software as a service. The Cyber Defense Index ranks the world’s 20 largest economies according to their collective cybersecurity assets, organisational capabilities and policy stances. It measures the degree to which they have adopted technology practices that advance resilience to cyberattacks and how well governments and policy frameworks promote secure digital transactions.
Looking ahead, the use of AI for both cyber defence and cyberattacks is expected to evolve over time. While AI tools in their current form may be particularly useful for creating more credible cyberattacks or exploiting deepfakes for social engineering, they could also be used to design new types of attack in the future. This highlights the need for cybersecurity professionals to exploit the benefits of technological advances such as AI to keep up with an ever-evolving cyber threat landscape and enhance cyber resilience. This is an area where, by international standards, at least some euro area countries appear to have room for improvement (Chart B, panel b). Dynamics in cybersecurity are essentially driven by an arms race between cyber defenders and threat actors – and AI is adding to the tools of both sides. It is currently difficult to assess who will gain the upper hand, and the momentum may well change over time. Nevertheless, given the potential for disruption if a systemic cyber incident occurs, it is important for financial institutions, as well as supervisors and regulators, to closely monitor associated developments.
With contributions from Nicola Doyle.
Case study conducted among 600 business owners. See Haan, K., “How Businesses Are Using Artificial Intelligence In 2024”, Forbes Advisor, April 2023.
See Kamalnath, V. et al., “Capturing the full value of generative AI in banking”, McKinsey & Company, December 2023.
Banking is expected to have an annual potential of USD 200 billion to USD 340 billion of added economic value (equivalent to 9-15% of operating profits), largely from increased productivity. See Chui, M. et al., “The economic potential of generative AI: The next productivity frontier”, McKinsey & Company, June 2023.
See, for example, Yong, J. and Prenio, J., “Humans keeping AI in check – emerging regulatory expectations in the financial sector”, FSI Insights, No 35, Bank for International Settlements, August 2021; “Artificial Intelligence and Machine Learning”, Discussion Paper, No 5/22, Bank of England, October 2022; Shabsigh, G. and Boukherouaa, E.B., “Generative Artificial Intelligence in Finance”, International Monetary Fund, August 2023; “Artificial intelligence and machine learning in financial services”, Financial Stability Board, November 2017, and as part of the FSB Work Programme for 2024,a follow up report on AI and their potential implications for financial stability. The EU is establishing the European Artificial Intelligence Board under the Artificial Intelligence Act and the European AI Office; the United Nations has established an AI Advisory Body; for the United States, see the Select Committee on AI and the Executive Order on the Safe, Secure, and Trustworthy Development and Use of Artificial Intelligence.
The overview aims to capture only the sub-fields of AI relevant for financial stability – it omits other sub-fields. Alternative approaches consider the two broad categories to be (1) weak AI or narrow intelligence (ANI), and (2) strong or general AI. (1) describes algorithms which are designed for a narrow task and try to mimic human behaviour. (2) defines algorithms which match and exceed human intelligence. There is a broad consensus that all AI technologies are currently weak AIs.
See Bommasani, R. et al., “On the Opportunities and Risks of Foundation Models”, ArXiv, Cornell University, 2021. The definition of foundation models is based on the author’s definition.
See Paunov, C. et al., “On the concentration of innovation in top cities in the digital age”, OECD Science, Technology and Industry Policy Papers, OECD, December 2019.
Self-supervision in machine learning utilises unlabelled data. Unlike labelled data, which provide indicators for predictions, unlabelled data lack these labels. Algorithms employing self-supervision initially discern patterns and structures in unlabelled data, then proceed to label the data themselves.
The pre-training on vast amounts of data is used to pre-calibrate the weights in the artificial neural network. The pre-calibration defines the “knowledge” of the artificial neural network (the foundation model) and can be used for generative AI models serving different specific downstream tasks.
See Bender, E.M. et al., “On the Dangers of Stochastic Parrots: Can Language Models Be Too Big?”, Proceedings of the 2021 ACM Conference on Fairness, Accountability, and Transparency, March 2021, pp. 610-623.
See Perez-Cruz, F. and Shin, H.S., “Testing the cognitive limits of large language models”, BIS Bulletin, No 83, Bank for International Settlements, January 2024. The analysis suggests that caution should be exercised in deploying LLMs in contexts that demand rigorous reasoning in economic analysis.
For further considerations, see among others Shabsigh, G. and Boukherouaa, E.B., op. cit.; Anderljung, M. et al., “Frontier AI Regulation: Managing Emerging Risks to Public Safety”, ArXiv, Cornell University, November 2023; Bommasani, R. et al., op. cit.; Yong, J. and Prenio, J., op. cit.; “Artificial Intelligence and Machine Learning”, Bank of England, October 2022; “Artificial intelligence and machine learning in financial services”, Financial Stability Board, November 2017; and Lorica, B. “Generative AI in Finance: Opportunities & Challenges”, Gradient Flow, August 2023.
This could entail the creation of not only synthetic data – which could make the training of new models more affordable – but also measured data.
In general, any AI output drawn from a data-based application depends on the quality of the data. If AI models, including machine learning and deep learning models, rely heavily on data that are biased, incomplete or contain errors, then the AI model will likely produce unreliable or biased results.
See Bommasani, R. et al., op. cit. The authors extensively discuss and explain the adaptable, flexible and scalable features of AI.
Some studies find factual errors in up to 46% of generated outcomes. See, for example, de Wynter, A. et. al., “An evaluation on large language model outputs: Discourse and memorization”, Natural Language Processing Journal, Vol. 4, September 2023.
Often referred to as AI’s black box problem (see “AI’s mysterious ‘black box’ problem, explained”, University of Michigan-Dearborn, 6 March 2023). This further relates to a more fundamental discussion on the trade-off between accuracy and explainability. More complex model structures may achieve more accurate predictions which are, however, difficult to explain. On the other hand, simpler models can be more transparent, although they may be less accurate.
AI system capabilities might depend on the concrete deployment of foundation models. Post-deployment enhancements, such as fine-tuning or allowing models to use external tools (like internet connection), can reveal new capabilities that are potentially dangerous or unexpected. See, for example, Anderljung, M. et al., op. cit.
This also raises the question of who is accountable in the event of a malfunction with unforeseen consequences.
Examples could include the gathering and documentation of information for internal reporting purposes, complaints management, legal assistance, HR processes, staff training, IT support lines, etc.
This also includes applications used by institutions to facilitate regulatory compliance (regtech), but supervisory authorities may also enhance supervision processes with AI (suptech).
Overall efficiency could be increased not only by financial institutions deploying AI but also by customers themselves relying on third-party AI advisors to find the cheapest financial products from different providers, for instance. This greater transparency could translate into lower margins for banks but higher overall efficiency.
Supplier concentration on the AI market can be seen as a result of rising oligopoly-like structures, which can already be observed e.g. on the market for cloud computing providers. See Narechania, T. N., and Sitaraman, G., “An Antimonopoly Approach to Governing Artificial Intelligence”, Vanderbilt Law Research Paper, No 24-9, November 2023 and Joy, M., “How Cloud Computing Companies Created an Oligopoly”, OnSIP, May 2021
See, for example, Bernanke, B.S., “Causes of the Recent Financial and Economic Crisis”, Testimony, Federal Reserve, September 2010, who defines the too-big-to-fail externality like this: “A too-big-to-fail firm is one whose size, complexity, interconnectedness, and critical functions are such that, should the firm go unexpectedly into liquidation, the rest of the financial system and the economy would face severe adverse consequences.”
According to an ESMA study, market participants in the euro area are increasingly using AI in investment strategies, risk management, compliance, data analysis and post-trade processes. See “Artificial intelligence in EU securities markets”, ESMA TRV Risk Analysis, ESMA, 1 February 2023.
See “Banks’ digital transformation: where do we stand?”, Supervision Newsletter, ECB, February 2023.
This survey does not capture generative AI tools based on foundation models.
From a financial stability perspective, concerns centred in particular on concentration risk arising from the limited number of vendors possessing the capabilities and technology to provide generative AI solutions, the scale of investment required (which some felt could favour large incumbents), data protection, the clustering of decision patterns, herding behaviour and cybersecurity.
See also in this context the EU’s Artificial Intelligence Act as a general legislative initiative to promote the uptake of AI and address the risks associated with certain uses of such systems.
See, for example, the article entitled “Towards a framework for assessing systemic cyber risk”, Financial Stability Review, ECB, November 2022, and “Systemic cyber risk”, ESRB, February 2020.
“Advancing macroprudential tools for cyber resilience”, ESRB, February 2023.
See “Staying ahead of threat actors in the age of AI”, Microsoft Threat Intelligence, 14 February 2024.
Phishing is a form of social engineering attack that aims to gather sensitive information by impersonating trusted sources or individuals. This can happen in writing or by phone, or a combination of both.